Machine learning aims to model a state, situation and/or phenomenon using historical data so that when new data comes in, it can be labelled with the learned system. One purpose of learning inductively from historical data is classification and the other is regression. For example, with a system that learns the data on past real and fake credit card expenditures, it is possible to classify whether a new expenditure is real or fake.
Sabancı University conducts studies on this subject in both Computer Science and Engineering and Industrial Engineering programs.
Funded Projects
- 2020-2023 TÜBİTAK Twinning Project (No:119E429): "A Deep Learning Based Hate Speech Recognition System: A Comparative Analysis of Turkey and Tunisia" B. Yanıkoğlu, R. Yeniterzi, A. Terzioğlu, İ. Arın
- 2020-2022 TÜBİTAK 1001 Project (No:119E429): "Classifying and Sorting Semantic Facial Attributes with Deep Learning", B. Yanıkoğlu
- 2020-2022 TÜSEB, "Synergistic miRNA Detection Platform for Cancer miRNA Therapies", Ö. Taştan
- 2018-2021 TÜBİTAK 3501 Project (No:117E140): "Discovery of Cancer Patient Subgroups with Functional Graph Kernels", O. Tastan
- 2014-2016 TÜBİTAK 1003 Project (No: 103E499): “Plant Recognition with Contextual Image Access Methods”, B. Yanıkoğlu.
- 2013-2015 TÜBİTAK 1001 Project (No: 103E062): “Developing and Implementing Handwriting Recognition Technologies to be Used in Smart Classrooms”, B. Yanıkoğlu
Faculty Members
Plant identification from photography is a difficult classification problem, with a high number of classes and high similarities between classes.
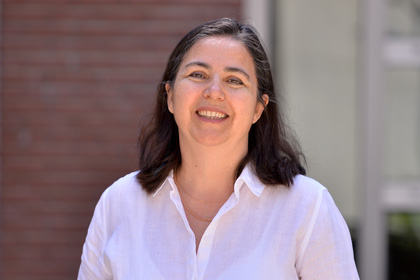
FENS Faculty Member
Research Areas
Computer vision & Machine learning: biometrics, document recognition, image retrieval
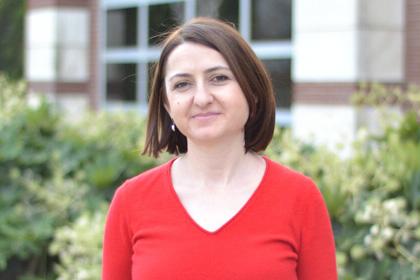
FENS Faculty Member
Research Areas
Machine learning, Computational Biology, Bioinformatics
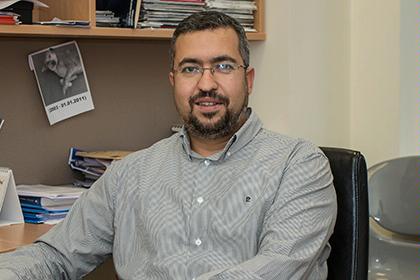
FENS Faculty Member
Research Areas
Research Interests • Decision Making Under Uncertainty • Heuristic Search and Optimization Techniques • Data Mining • Decision Support Systems • Bioinformatics • Innovation Management
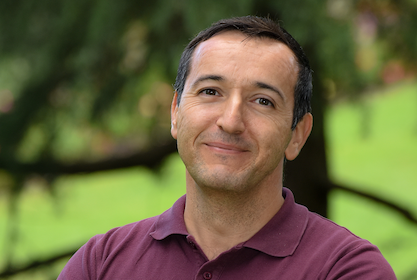
FENS Faculty Member
Research Areas
Computer vision; digital image analysis; remote sensing; precision agriculture; biomedical image processing.
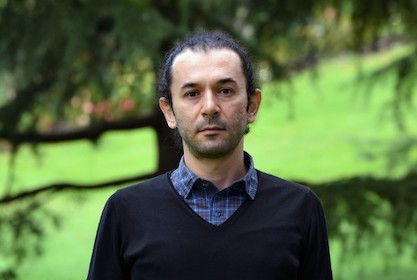
FENS Faculty Member
Research Areas
Automated Program Repair; Automated Fault Localization; Fix Pattern Mining; Mining Software Repositories; Machine Learning and Natural Language Processing for Software Engineering; Empirical Software Engineering.
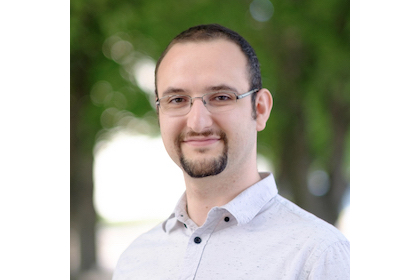
FENS Faculty Member
Research Areas
Data Science, Machine Learning, Computational Social Science, Network Science, Complex Systems